Data drives decision-making, now more than ever. In fact, data-driven decision-making has become so essential to all industry sectors that global predictive analytics revenues are expected to reach $22 billion in 2026.
The explosion in data has transformed health care, too. Before the pandemic, researchers calculated that patients generated 80MB of data a year. That figure has certainly been surpassed. Data comes from everywhere, now — including personal health information, disease registries, population health statistics, wellness apps, and wearable devices. It includes insurance, geographic, and financial data.
All of that information represents a powerful tool for healthcare administrators — if they can harness it, that is. Applied data can improve medical care, make administrative processes more efficient, save money, and reduce burnout among clinical staff. Increasingly, hospitals draw from data analysis and predictive modeling to support unbiased and evidence-based strategies and decisions.
What Is Data-Driven Decision-Making?
Data-driven decision-making (DDDM) uses information that has been gathered, modeled, and analyzed to gain an understanding of specific business challenges and to support effective solutions. Data must be reliable, accurate, valuable, and pertinent, which means it must be timely, cleaned of errors, and applicable to the question under consideration. The goal of DDDM is to take the guesswork out of decision-making. Business leaders who make decisions based on data analytics say that their companies have experienced improved efficiency and financial performance.
Advantages of data-driven decision-making include:
- DDDM makes data usable. Companies can surface relevant information about their products, operations, and customers through data analytics.
- Data provides insight. DDDM makes designing strategies more concrete. Leaders who have access to accurate data can gain insights into the company’s operations that in turn drive decisions.
- Data can be qualitative or quantitative. Qualitative data may be customer feedback or, in the case of health care, a nurse’s notes. Quantitative data comprises statistics or numbers. This could be billing information or ZIP codes. Both types have value.
- Decisions are transparent. Companies can point to the data underlying their strategies, which can increase employee support.
- Companies are more agile. With the proper data analysis tools, leaders can make decisions faster and more effectively and with less ambiguity.
As illustrated here, data-driven decision-making has many benefits. However, business leaders must have a complete understanding of what data-driven decision-making is and its capabilities to make fully informed decisions. Pitfalls of DDDM include:
- Misinterpreting the data. Using data to justify decisions already in play or misunderstanding what the data is revealing are common pitfalls of data-driven decision-making. This misinterpretation can lead to poor outcomes.
- Asking the wrong question. Even high-quality data can lead to poor results if companies use the data to answer the wrong question.
- Using poor data. Companies need to invest in data management tools and ensure that data analysts and data scientists rigorously cleanse the data of factual errors, redundancies, and other issues before it is used.
- Using too much data. The term big data refers to the vast oceans of data that users, companies, industries, governments, and other entities generate on a daily basis. While big data has its uses, managing too much data in certain cases eats up computing and financial resources and does not yield enough actionable information to justify the cost.
Types of Data Analytics
The following four types of data analytics are used for data-driven decision-making.
Descriptive Analytics
In simplest terms, descriptive analytics describes the behavior of customers or clients and illustrates their behavior.
In marketing, for example, this may be information such as how long a customer browsed a website, what pages they stayed on longest, what they clicked on, and what they purchased. It can include whether they abandoned a shopping cart without completing a purchase, whether they searched from a mobile device or a desktop, and even what day they shopped.
In health care, descriptive analytics comprises historical patient data. This data includes patient visits, prescriptions, surgeries and other treatments, and patient outcomes. It also includes payment and billing, geographic data (which can be used in population health metrics), and more.
Once business leaders understand the behavior of their customers or patients, they can tailor their decisions for the best outcomes. For instance, historical patient data could support preventive care initiatives or predict risk factors for certain diseases.
Diagnostic Analytics
If descriptive analytics tells companies what happened, then diagnostic analytics explains why it happened. Also called root cause analysis, diagnostic analytics helps business leaders understand why the company or organization got the results it did. For instance, diagnostic analytics can help uncover why sales trended up or down by analyzing what customers bought — or did not buy — during a specific period.
Diagnostic analytics in health care can be used to gain understanding at the patient level up through the organization level. Clinicians, administrators, and healthcare executives can use the information gleaned from diagnostic analytics to understand many complex challenges in medical care.
These issues are so complex that diagnostic analytics requires artificial intelligence (AI) running on powerful computers to analyze the vast quantities of data involved at speeds much faster than a human could manage. AI and machine learning use algorithms not just to collect data but to find connections and speed up decision-making.
For instance, AI can read research articles, test results, clinical trials, and other data, and compile all of this information to speed up drug development. AI has a better success rate than radiologists in identifying false positives in mammograms, and accuracy that is comparable to that of radiologists in detecting cancer.
This combination of AI and diagnostic analytics is revolutionizing health care. AI can parse vast quantities of patient electronic health records (EHR) and supply data to physicians and nurses to help drive their decision-making. This capability is spurring a movement toward clinical decision support and evidence-based care, changing how doctors and nurses make data-driven healthcare decisions.
Predictive Analytics
Predictive analytics uses current and historic data to develop a model of predictive behavior. Data scientists use many techniques, including statistical analysis, machine learning, and data queries, to determine the likelihood of an event. This likelihood is represented as a numeric value, or score. Predictive analytics is a highly complex process. The financial services, insurance, scientific research, and of course healthcare industries all make use of predictive analytics. The goal of most predictive analytics in business is to mitigate risk. One example of this is the way insurance companies use predictive analytics, actuary science, and other data science to reduce underwriting costs.
Successful applications of healthcare predictive analytics include:
- Patient treatment. In health care, predictive analytics has successfully identified effective patient treatments, estimated patients’ disease risk, and helped clinical staff prevent patient deterioration. It can be used to identify risks due to socioeconomic status and ZIP code, some of the social determinants of health. The goal is to improve patient outcomes due to more effective medical treatment and preventive care.
- Patient data management. EHR systems can be highly effective tools for collecting patient care data. However, they can be difficult to use, as they include clinical, billing, and other patient information. Misuse of EHR systems can cause safety issues. The use of predictive analytics can relieve staff of some of the burden of information overload from EHRs.
- Billing and revenue cycles. Predictive analytics can be a useful tool in the revenue cycle. Hospitals use modeling to improve insurance reimbursements and patient payments. Administrative, finance, and accounting staff can gain a better picture of a hospital’s or provider’s financial picture and delegate resources accordingly.
- Workforce optimization. Hospital staffing shortages can lead to burnout among clinical staff and result in medical errors. The pandemic spotlighted these challenges. Predictive analytics can help hospitals identify when they will need to ramp up staff so they can be prepared for a surge in patients. Data includes bed capacity, payroll data, and nurse-to-patient ratios.
Prescriptive Analytics
Once leaders know what happened, why it happened, and what is likely to happen, they turn to prescriptive analytics to help identify a plan of action. In essence, prescriptive analytics is used to determine the best possible solution based on the data. Businesses use prescriptive analytics to support their decisions related to day-to-day actions as well as short-term and long-term goals.
As with the other data analytics processes, prescriptive analytics uses AI and machine learning to parse the vast quantities of data available and recommend actions based on algorithms. This can be automated, as in the case of delivery route generation.
Prescriptive analytics can help reduce costs and optimize health care. Consider the following examples.
- Claims data. An insurance company can use claims patterns to justify changes in premiums or add rewards for preventive care.
- Logistics. Everything from transporting patients to ordering supplies can be modeled using data analytics, and hospitals can use prescriptive analytics to determine which logistics procedures and policies work best.
- Radiation treatment. Oncologists can use modeling data to determine the best radiation dosage for patients.
- Staff planning. Hospitals can determine staffing levels based on data models.
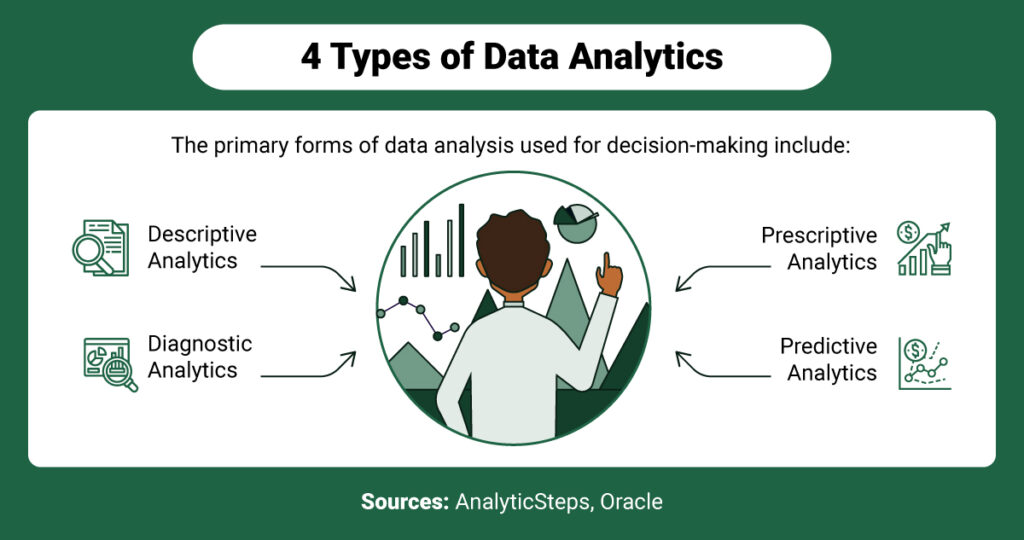
Data Analytics Tools
Data scientists use a variety of mathematical and analysis tools to manage and model data, submit queries, and parse the results. Administrative and executive staff use a variety of tools, as well. These tools are software applications that present the data in visual formats that enable managers and employees to more easily understand it for data-driven decision-making.
Data Science Tools
Data scientists use programming tools and statistical libraries to perform their analyses. These include SAS, Spark, Java, BigML, and MATLAB. These tools are used to manage and model data, write algorithms and formulas, generate graphs, and perform other functions. They can be used to generate natural language queries, which allow computers to parse human language. They can also be used to develop fuzzy language or neural net applications, which are used in machine learning.
Interactive Dashboards and Data Visualization
Interactive dashboards make it easier for users to visualize data and make decisions based on all the pertinent information. The dashboard updates in real time, so administrators and other decision-makers have the most valuable information to work with. Many dashboards include alerts, report-building functions, and forecasting capabilities.
A healthcare data dashboard will include financial, patient, billing, human resources, and other operations data. It will let administrators see how all of these factors interact. Hospital leaders can use this data to support short-term and long-term goals.
Business Intelligence
Healthcare business intelligence (BI) uses data analysis to identify ways in which healthcare providers can improve their overall business position in a market. Healthcare BI integrates with medical and patient data, but uses that data to find ways to improve an organization’s financial health and operational efficiencies. These tools can be used to take a look at patient outcomes in view of improving a hospital’s risk profile. It can combine patient and staff safety data. A business intelligence tool will also include data visualization through a healthcare data dashboard.
Data-Driven Decision-Making in Health Care
Healthcare decision-making puts patient care front and center. However, as seen in the examples above, excellent patient care involves all aspects of the patient’s experience and not just their medical treatment. That is why patient care metrics include patients’ satisfaction as it relates to billing, appointment scheduling, and prompt treatment. All of these metrics are included in the data that administrators use to make daily and long-term decisions.
When big data is managed correctly (collected, cleaned, stored, and parsed) and interpreted accurately, it is extremely useful for improving patient satisfaction and outcomes. In turn, patient outcomes drive the financial health of hospitals and other organizations.
Five key benefits of data-driven decision-making in health care are:
- Supports clinical treatment decisions. The effectiveness of big data combined with AI modeling supports clinical treatment, whether that is by speeding up drug development or increasing the rate of accuracy when identifying tumors in cancer scans.
- Reduces disease risk. Decision-makers can draw from vast quantities of population health metrics to identify entire communities at risk of chronic disease. Healthcare providers can allocate resources to improve preventive care. Additionally, the evolution from a fee-for-service to a value-based care model prioritizes disease prevention over treatment.
- Improves efficiencies. Providers are turning to chatbots to speed up the appointment process, relieving administrative staff of time-consuming tasks. Healthcare data can be used to manage staffing levels, identify billing inaccuracies, and streamline hospital operations.
- Reduces costs. According to the public health foundation the Commonwealth Fund, the U.S. spends more per capita on health care than any other wealthy nation, but ranks at the bottom in health care outcomes. DDDM can help hospitals identify their inefficiencies and offer more cost-effective care.
- Empowers patients. The popularity of smartwatches and other consumer health devices is an example of consumer demand for health data. Combining consumer health data with EHR data and providing that information to patients can educate them on their disease risk and their opportunities to take charge of their own health goals.
Data-Driven Decision Resources
The following resources describe how data-driven decision-making in health care can transform an organization. They touch on the advantages of taking a data-driven path, as well as the many challenges of making the shift, including the data quality, legacy systems that are not optimized for data, and the need for investment in technology and training.
- The Commonwealth Fund, “Consumer Choice in U.S. Health Care: Using Insights from the Past to Inform the Way Forward.” — Healthcare experts analyze consumer behavior to identify how patients make healthcare decisions, the better to understand what they value.
- Forbes, “How Healthcare Organizations Can Become Data-Driven.” — Many healthcare providers do not know where to start when it comes to reorganizing into a data-driven organization. The first step is to get buy-in from all stakeholders on this important change.
- Harvard Business Review, “Fixing Data Overload in Health Care.” — Data needs to be managed before it can be analyzed. Healthcare organizations need to segment and integrate their data before getting valuable information from it.
- Pew, “Most Americans Want to Share and Access More Digital Health Data.” — A survey of American consumers reveals that people want access to their health data on their devices but are also concerned about data security and privacy.
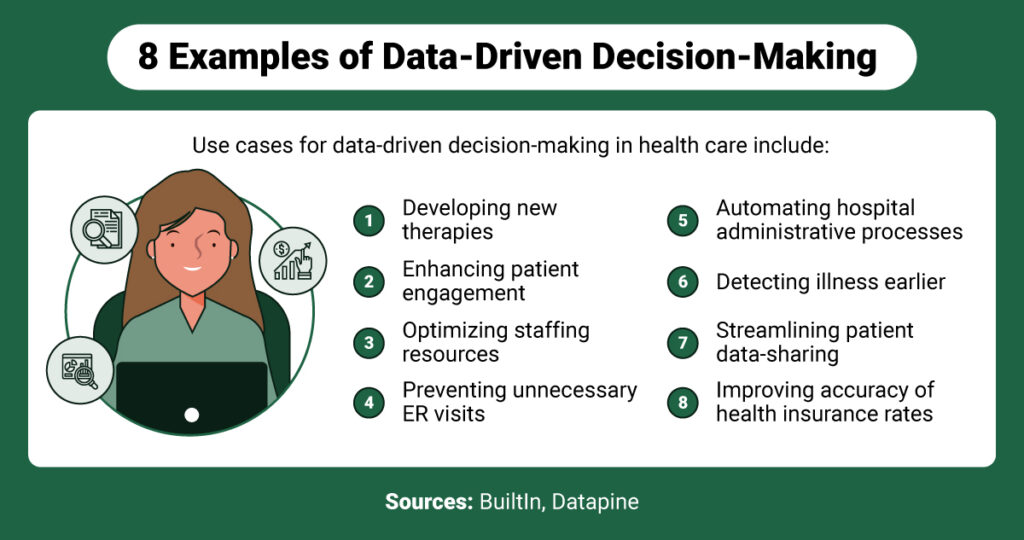
Data-Driven Strategies for Health Care
The primary goals of implementing a data-driven strategy include improving healthcare operations, reducing costs and increasing revenues, and optimizing workplace performance. In practical terms, that means improving patient care, ensuring that insurance reimbursements are timely, and making sure that clinical and administrative functions are appropriately staffed and employees are not overworked.
Examples of Data-Driven Decision-Making
Many healthcare organizations have put data-driven decision-making into practice. All aspects of a hospital’s operations are fair game for improving processes and measuring outcomes. The following are examples of how data analytics is changing decision-making and operations.
- Personalized medicine. Scientists are looking at the collection of high-quality data from EHRs and other sources as a way to develop personalized treatments for cancer and other diseases. Because data is collected with each appointment, doctors can track the effectiveness of treatments, and then add that data to the knowledge base.
- Patient engagement. Healthcare marketing teams can analyze data to determine the best ways to communicate with specific patients and caregivers and take action based on that analysis. For instance, they may provide targeted email newsletters to parents of their pediatric patients or to elderly patients based on their needs.
- Personnel management. Human resources dashboards provide administrative teams with a clear picture of staffing needs and effectively predict when demand will rise and fall. These dashboards work in concert with scheduling dashboards and can anticipate when operating rooms and intensive care units will be the busiest.
- Medical errors. Clinical staff may be vulnerable to information overload. They may be busy due to understaffing. Data analytics can potentially catch medication errors by flagging discrepancies in a patient’s EHR.
- Early illness detection. AI and machine learning are effective tools for identifying cancer and other illnesses at very early stages.
- Billing errors. Data analytics can be used to manage the billing cycle and help uncover waste or fraud.
- Patient data-sharing. As anyone who has ever been handed a clipboard at the doctor’s office can confirm, patient data collection is redundant and inefficient. Additionally, patient data is protected by federal regulations. However, sharing data via EHRs and on disease registries with identifying data stripped out can be a powerful tool for developing new therapies and improving population health.
Data-Driven Strategies
The healthcare industry has been dealing with the challenge of managing and utilizing its data for decades. When the Affordable Care Act was enacted in 2010, it included a provision for EHR applications. Many legacy health records stored on paper had to be scanned into new systems. The quality of that data may be poor, redundant, or unreadable.
Hospital administrators understand that data analytics can be used to clean up that data and make it usable. The problem they may have is that they do not know how to get started on this monumental task.
The following are strategies for implementing a data-driven mindset.
- Eliminate data silos. Data silos prevent data in different systems from “talking” to each other. However, data can be more powerful when it is combined with other data.
- Assess analytics maturity. Measuring an organization’s capacity for an analytics strategy is a crucial step.
- Align goals with analytics. Do not use analytics to support existing goals. Instead, establish goals first, and then use the data to drive how to achieve them.
- Develop standards and governance. The pillars of data analytics include trust, accountability, transparency, collaboration, risk, and education.
- Create stakeholder-driven applications. EHR systems have a great deal of potential. However, when this technology is imposed on clinical staff, it can add to their stress. Stakeholders should be involved in choosing technology.
- Democratize data. Allowing all stakeholders to have access to data, including patients, is part of the democratization of that data. This has been linked to better patient outcomes.
Data-Driven Strategies: Resources
The following resources provide a road map for moving toward a data-driven decision model. While the task is daunting, experts have broken the process into a series of actions.
- Hartman Executive Advisors, “8 Steps to Becoming a Data-Driven Healthcare Organization.” — Understanding the data a company has and the challenges they are attempting to solve is an important launching point for the move toward DDDM.
- HealthCatalyst, “Four Elements That Bridge the Gap Between Using Data and Becoming Data-Driven.” — Data governance, data security, data literacy, and a commitment to a single source of data truth are the marks of a successful data-driven model.
- TIBCO Blog, “How to Become a Data-Driven Healthcare Organization in 7 Steps.” — Eliminating data silos is one of the most important steps in extracting value from healthcare data.
Data-Driven Decision-Making Is Changing Health Care
Data-driven decision-making has revolutionized health care. Data analytics provides doctors, clinical staff, and administrative managers with the information they need to improve health care from all angles, without resorting to hunches or biases. They can make informed decisions that are aligned with and support their business strategies. Patient outcomes and satisfaction, quality of care, and increased efficiencies are the positive results of a well-managed data operation.
Hospital administrators and healthcare providers who commit to a data-driven process and follow the steps that experts recommend (maintain high-quality data, include all stakeholders, and invest in resources) can expect to see sweeping benefits across all departments.
Infographic Sources:
Analytics Steps, “What Is Data Analytics and Its Types?”
Built In, “11 Big Data in Healthcare Applications and Examples”
Datapine, “21 Examples of Big Data Analytics in Healthcare That Can Save People”